American Mobile Bitcoin Casinos 2024 - Top Bitcoin Casino Apps
Online casino guide
Not all online casinos are rigged. While there are a few that rig their software or cheat, the vast majority are not. Every bet has certain odds of winning, and the payout odds are always less than the odds of winning, but this does not mean the results are not random. Just make sure you do your research.
An online casino is an establishment that offers a variety of casino games for gamblers to enjoy. They also go by other names such as virtual casinos or Internet casinos. These casinos allow people to play their favorite casino games over the internet. Many people enjoy playing at online sites because of the convenience and flexibility of the site. To learn more about the benefits of online casinos, read on. Here’s a brief overview of the industry. Let’s get started!
First, online casinos use different software to operate their games. Some use their own software, but most use third-party developers. Some websites use a single provider, while others utilize several different providers. In this guide, we’ll examine the best software providers used by online casinos to make their games. This information is especially helpful for those looking for a new online casino. You can also sign up for their newsletters to receive important updates about their latest promotions and new features.
The second option is to look for an independent testing lab. Most online casinos will offer certificates from this organization. A reputable testing lab will have a seal that ensures the games are fair. If an online casino is not certified, you should avoid it. You can find independent laboratories by checking their website’s licensing requirements. These labs are the best resources to find out if an online casino is legitimate. You can also find information on the licenses of online casinos.
Minimum deposit and bet
The minimum deposit differs but it is usually 1 m฿, around $8. Your bitcoin balance can then be bet in parts as small as 0.001 m฿, which one is about $0.008c.
Provably fair gambling
Definitely fair gambling does not depend on cryptocurrency, it's same as easy for government currency operators to implement.
Crypto casinos for high rollers
Bitcoin gambling is a high rollers dream come true. There exist no limits to deposits and withdrawals, any one that are instant and free. Existence anonymous offers you privacy and security.
Bitcoin casino bonuses
Few crypto casino bonuses are a fine way to get started and enhance the enjoyment you can get from a deposit. still if you are unlucky at the start, you will be further in profit due to your bonus!
Online casino selector
There are so much casinos and they all looks the same, so how do you choose best one to join? In fact they're not the same, any have better customer service, but terrible payouts, some have greater bonuses but a awful live dealer zone.
Mobile casino games
Most bitcoin casinos has a mobile version, where you can play all games provided on the desktop version. It is even regularly updated, so gamblers can make fun their favorites in moments they're away from home.
Best Online Casinos
Bet365 Casino
Bet365 is one of the world’s leading online gambling companies. Bet365 offers a wide range of betting opportunities, from sports betting to casino games, poker, and even financial betting.
The company was founded in 2000 by Denise Coates, who started the business in a portable building in Stoke-on-Trent in the UK. Bet365 has since grown to become one of the largest private companies in the UK, with millions of customers worldwide.
Bet365 is particularly well-known for its sports betting platform, where users can bet on a variety of sports events, from football and horse racing to cricket, tennis, and more. The company is also recognized for its live streaming service, which allows customers to watch and bet on live sports events through their website or app.
It’s important to note that the availability of bet365 services can depend on your location, as online gambling laws vary by country and region. Always make sure to comply with your local laws and regulations when engaging with online betting platforms.
Logging into your bet365 account is straightforward and should only take a few moments. Here’s a simple step-by-step guide:
1. Open your web browser and navigate to the bet365 website.
2. Once you’re on the home page, you will see a ‘Bet365 Log In‘ button or link usually at the top right corner of the screen.
3. Click on ‘Log In’ to open the login form.
4. Type your Username or Email and Password into the corresponding text boxes. Make sure you input them correctly as they are case sensitive.
5. After you’ve entered your login details, click on the ‘Log In’ button to sign into your account.
6. If your details are correct, you should be logged into your bet365 account and ready to start using their services. If you have trouble logging in, it could be because you’ve entered the wrong username or password or your account is locked due to suspicious activity. If you forget your password, click on the ‘Lost Login?’ link to start the process of resetting it.
Remember, it’s crucial to keep your login details confidential and secure. Don’t share them with anyone, and avoid logging in on public computers to protect your account.
Please note that online gambling is not legal everywhere, and the availability of bet365 varies by location. Always make sure to comply with your local laws and regulations.
Latest News
Cleopatra Slot Casino Bonuses and Promotions
BetBeard Casino offers 2,500+ casino games and video poker
Best Casino Affiliate Programs
Our target is to present the most reliable information to ardent online bitcoin casino players. We are focused to bringing our audience trustworthy facts on online crypto casino gambling to advise and provide players in setting the best of terms in this advantageous industry. On this web, you will find updates on what is attractive in the gambling industry:
- bitcoin gambling
- 747 live casino
- trusted reviews on crypto casinos
- casino bonuses and promotions
- must try bitcoin slot games
- all about online sports betting
- arzemju kazino
All information depend in this web is exactly for information purpose only. Readers and online casino players are proposed to attentively evaluate and familiarize personally with the policies of the online bitcoin casinos they choose to participate in. Reginnovations.org does not hold any responsibility for any losses caused by gamers at any of the gambling sites stated in any of our blog contents.
About Us
Our Mission
Our mission is two pronged:
- First, we focus to help as much people as possible to reach the best and most useful gambling site for their own personal requires.
Because of that we rank the leading gambling sites in a class of different categories. We regularly update our rankings to check they are current and accurate.
- Secondly, we point to make Reginnovations.org the single top resource on the internet for everything related to online bitcoin gambling.
We are already far on our way due to this. The site already includes a large quantity of information that contains a wide range of gambling related themes. We have more information expected, and are persistent to grow the site.
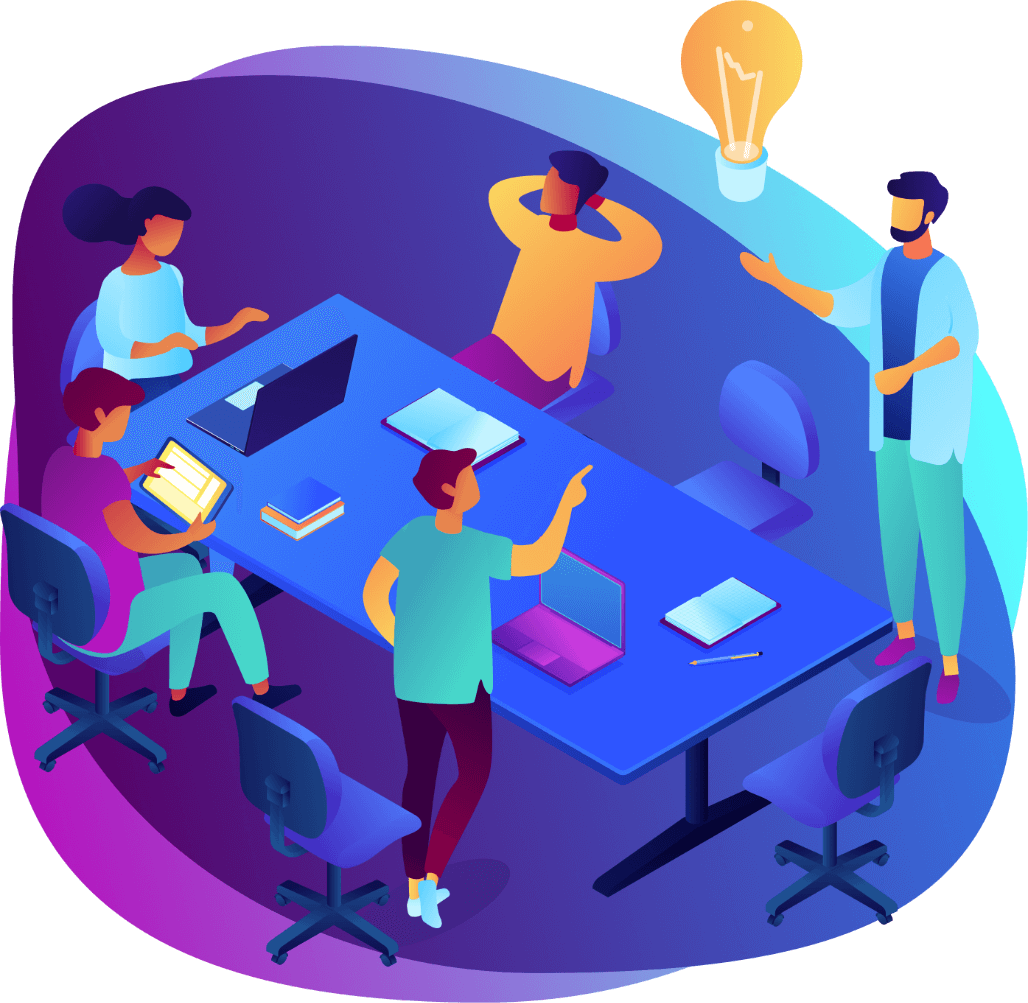